WARNING: I’m absolutely getting into stuff I don’t fully understand today and am likely to make mistakes, mischaracterizations, and boneheaded failures of comprehension. Here’s an artists rendition of me using advanced statical models:
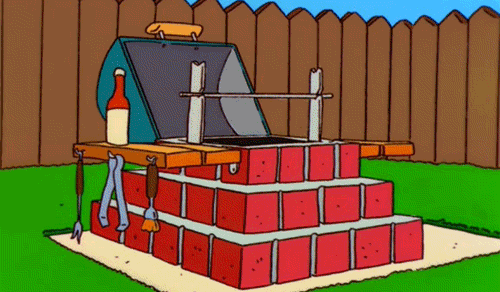
So please, feel free to correct, discuss, and/or gently disagree with me. You can do so in the comments section below! It’s fun!
But let’s back up a bit. One of the things that writing pieces like last week’s post on Jaylin Davis has made crystal clear is the yawning gap between public facing major league data and public facing minor league data. When I want to talk about the Mauricio Dubon’s pitch selections, thanks to his major league service time and the Statcast data on Baseball Savant, I get things like this to work with:
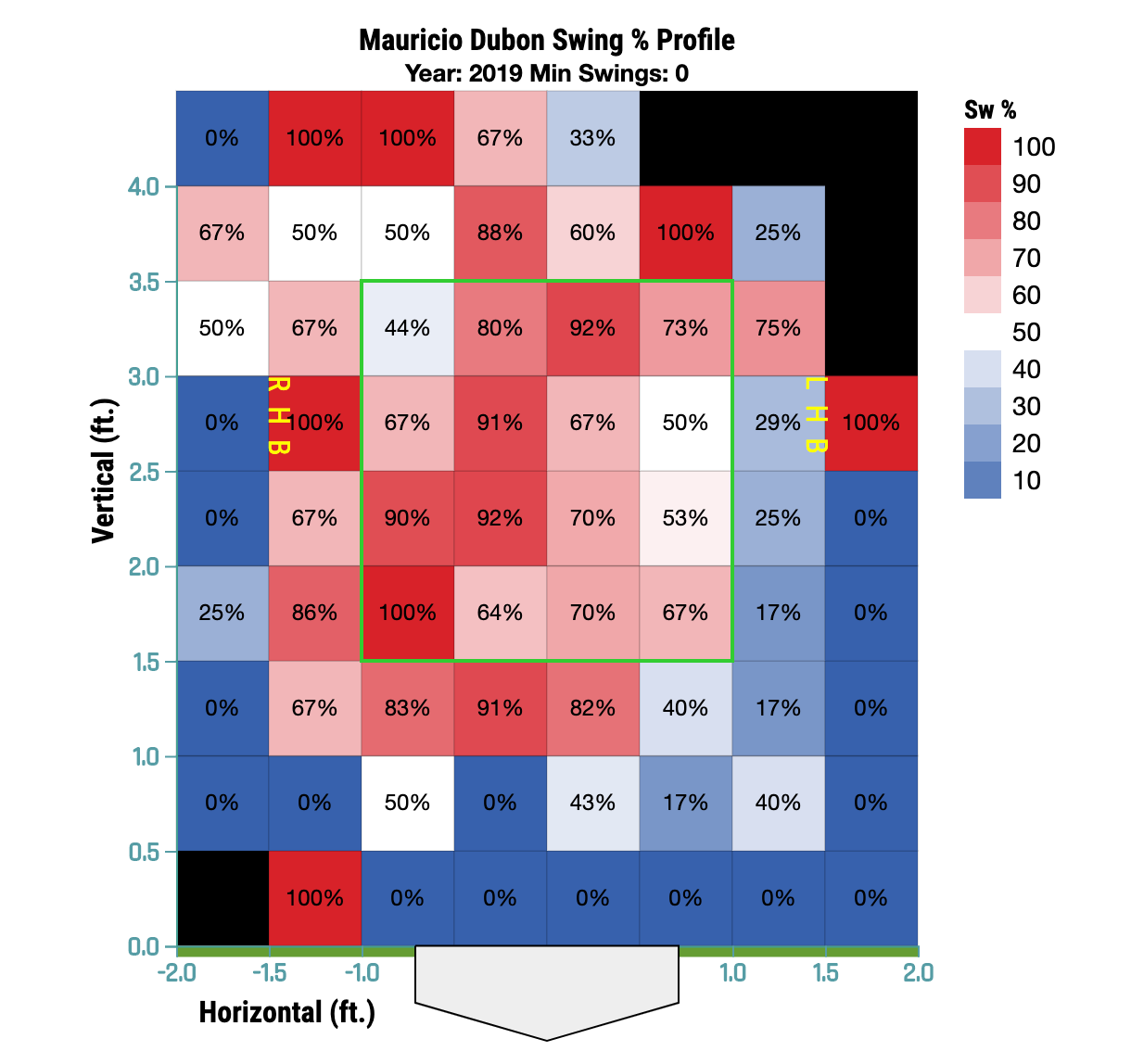
But when I want to talk about Luis Toribio’s pitch selection, I’m reliant on things like this scouting report from Baseball America’s “Prospect Handbook”:

The disparity is pretty sharp! Now this isn’t true for the teams themselves as nearly all minor league facilities now have Trackman systems set up and front offices are looking at data every bit as sophisticated for their rookie complex players as for their major leaguers (as they should!).
Most of that data is (probably rightfully) hidden away from us. Though we’re starting to see behind the curtain in drips and drabs. If you listened to the club’s radio broadcasts (back when such things existed) you’d occasionally hear the broadcasters refer to exit velocity or flyball distance on particularly impressive blasts, or even things like chase rates when discussing a player’s season. Baseball America is also publishing some of this data in their articles or scouting reports. Fangraphs took the first big step beyond anecdotal data when they started publishing average and maximum exit velocities for prospects which has been a huge boon. More off the beaten trail, Rotowire is now publishing “hard hit rates” for players at full season levels (good news for Zach Green fans!), and Prospects Live is publishing average fly ball distance for nearly all prospects on their team lists (“nearly” because there are weird gaps, as for instance neither Will Wilson nor Chris Shaw has any recorded fly ball distances from last year). Thus we know, for instance, that Heliot Ramos average fly ball distance last year was 306’ in the Cal League and 310’ in the Eastern League. Or that Jaylin Davis posted an extraordinary 338’ on average last year on his flyballs. Pretty good!
All of this is moving our understanding of minor league performances, but still, it’s giving a very incomplete picture. As Connor Kurcon has pointed out, average fly ball distance can give us very misleading information, as a hitter who hits a 200’ fly ball and 400’ foot fly ball is far preferable to the one who hits two 300’ fly balls.

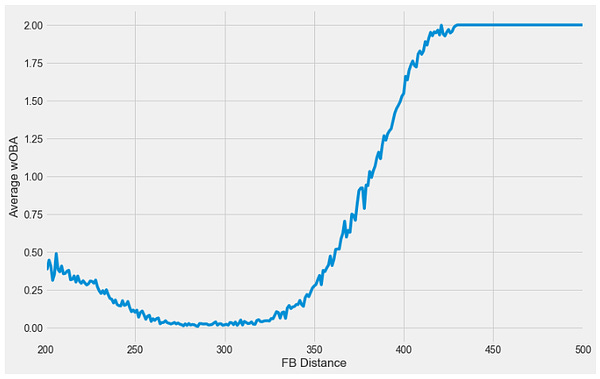
Fortunately, the internet is full of astoundingly brilliant young minds building models that make sophisticated interpretations of the data we do have. One of those brilliant folks is Jordan Rosenblum over at The Dynasty Guru who has, among other things, created a plug and play tool that interprets available minor league statistics and provides Major League Equivalents (MLEs) for them. MLEs have a long and somewhat controversial history. Bill James thinks they are one of his greatest contributions to SABRmetrics, while Tom Tango “hates how they are used” but acknowledges them as a useful tool. As Tango notes, MLEs obviously have issues with selection bias and sample size (only players who actually make the majors can be part of the sample behind the modeling) and they don’t understand most forms of context — players working on mechanical changes, players playing through minor injuries, all manner of development work. What they do understand is a player’s performance in the context of their age relative to their level, and the league and park offensive environments. They also absolutely are NOT meant to tell you what an 18-year-old Marco Luciano would do if he were bizarrely promoted to the majors today. They simply take a historical performance, filter it through historical data and quantitative context, and produce an equivalence of what that stat line would read at the major league level.
Basically, they’re a much more sophisticated version of your own eyeball test — where you look at Heliot Ramos’ line in the Cal League, take note that he was just 19 and say “Hubba Hubba!” Yeah, they’re that, only with the “Hubba” translated into Latin.
So with that caveat — they don’t mean everything, but they’re more than nothing — let’s dive in, shall we? Rosenblum’s MLE calulator (which is the only freely available calculator on the web) produces MLEs for batting average, on base percentage, slugging percentage, BB and K rates, and also for weighted on base average (wOBA). wOBA, originally created by Tom Tango to assign a “proper” weighted value for each type of hitting event (how much more valuable is a double than a walk or a groundout, etc) plays a major role in the calculation of Fangraphs’ WAR. (Baseball Savant has now gone a step further with expected wOBA, or xwOBA, which is built using Statcast data on contact quality from each at bat and has proven to be a remarkably predictive tool). Two features of Rosenblum’s calculator make it particularly useful:
It can produce a single MLE outcome from multiple different inputs (so you can plug in stops at several different levels for one player and get one integrated MLE otuput); and
In addition to the 2019 MLE it also models a projected outcome for the player at his major league peak performance. This is what I’m going to focus on.
I plugged 52 different Giants’ prospects into the calculator using their 2019 minor league season totals across all levels. I wanted to throw a broad net rather than just look at the top prospects, in part because I wanted to see how those elite prospects performed within the context of their less renowned teammates, and I also wanted to see if we could identify any pleasant surprises or potential sleepers using this tool. You can see the full outcomes for all of those players at this Google doc (please let me know if this isn’t working correctly).
But for now let’s focus on that expected peak outcome column (xwOBAp). The first thing I wanted to do with this data was simply plot out where the greatest talent in the system was in terms of age. We know that getting to the majors at a young age correlates very strongly with peak value. The graph below lays out the Peak Expected wOBA for all players on the Y axis, with their birth year on the X axis (I know it looks weird to see players were born in 1997.5, but it took me hours to get R to produce this result, so please have pity on me and go with it!) The line in the middle represents the 2019 American League wOBA for the average player (.320). Players above that line, by this projection model, stand a strong chance of producing a major league average performance at their best, while those near or under that line could still see useful careers as depth players or up and down guys.
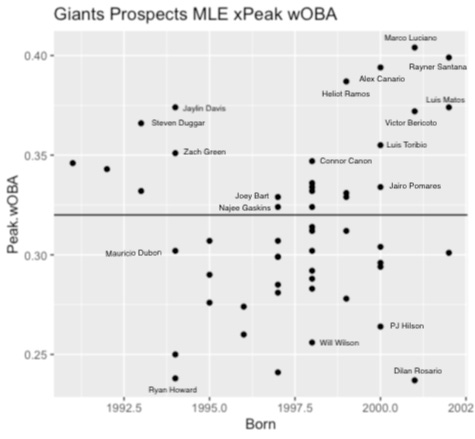
Not too surprisingly, this confirms what we all think about the Giant’s current system — that the impact talent is in the youth. Congregating in the far upper righthand corner are both the system’s youngest players and those projected to have the greatest peak performances in the system. Part of this, of course, comes from the fact that it’s much easier to dominate low levels of competition and pile up great stats, and thus a player like Rayner Santana gets an outsized projection result here that doesn’t necessarily reflect his scouting report. But the model believes that showing that much power at 16 in a league where players don’t normally hit double-digit Home Runs is really meaningful. So perhaps we should be talking about Santana much more than we do?
Quite logically, the other group that projects to produce above average MLB stats are the guys closest to the majors — all of those dots grouping together in the upper left-hand quadrant played in AAA last year. This presents something of the opposite problem from Santana. Unless one believes that ALL of Jaylin Davis, Steven Duggar, Austin Slater, Joey Rickard, Zach Green, and Chris Shaw are WELL above average major league hitters, then we might have to presume that the 2019 Pacific Coast League has broken this calculator. Still even within that context Jaylin Davis’ 2019 is pretty extraordinary — and we should remember in looking at this that more than 500 of Davis’ PA last year came outside of the PCL and 250 of them were in the much more difficult hitting environment of the Southern League (AA).
Both of these things show up as well if we look at the data in another way. Here the data is plotted with the 2019 MLE wOBA on the X axis and the expected Peak wOBA on the Y axis:
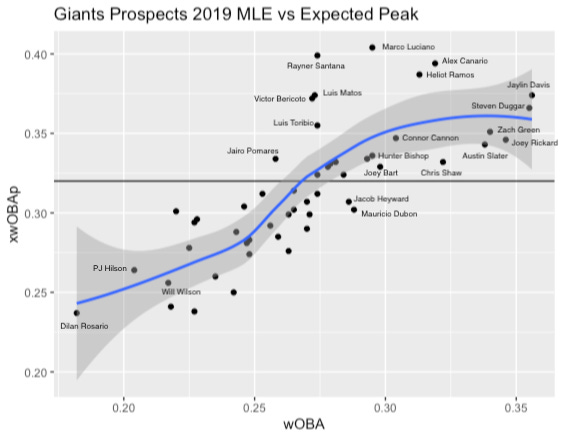
Not surprisingly the youngest players are the furthest off the mean line, with their projected peaks being dramatically separated from their 2019 MLEs. The AAA players (all of whom cluster on the smoothing line on the far right of the graph) basically show up as “near finished” pieces — their 2019 equivalent stats are nearly identical to their expected peak — with only Davis’ projected outcome really separating. Though while looking at Duggar’s MLE, you might want to keep this picture in mind:
Other things worth noting in these charts:
The model loves Alexander Canario in a really surprising way. I would have thought Canario’s extreme strikeout rates in the NWL (combined with being not overly-young for his levels), would have caused the calculator to ding him significantly. And yes, his 2019 MLE strikeout rate calculates to a mind-melting 46%! But even with that, the model projects him to have the third highest peak in the system (.394 xwOBAp) and more surprisingly, his 2019 wOBA was the highest in the system of any non-PCL player, and seventh highest overall (.319)! Wow!
The model believes Heliot Ramos’ 2019 was REAL — and significantly more predictive of future impact than, for instance, Joey Bart’s. Ramos’ bat really does look like it could play from any spot in the OF based on this particular view, though his projected peak is nearly as disparate from his 2019 MLE as guys like Luciano and Santana.
Joey Bart’s bat doesn’t project to be as explosive as some of the OF kids but providing solidly above average wOBA out of the Catcher position still gives him an excellent expected outcome.
Positive surprises include Connor Cannon, who also shows up extremely well in Fangraphs’ exit velocity data with a maximum event of 116, highest in the system last year. Cannon’s biggest flaws are absolutely no defensive value and an atrocious health record. But with the universal DH seemingly not far away, this gives a glimpse of a surprisingly solid outcome if Cannon can keep his health together.
Another surprising outcome — look at Najee Gaskins go! The 20th round pick from St. Cloud State is maybe the biggest outlier here. Despite being quite old for rookie league level, Gaskins has a believer in this calculator, just getting over the .320 average line with his expected peak wOBA of .324. Watch for him as a sleeper — maybe there’s a Juan Perez’ type outcome for this kid?
Given that the PCL environment clearly broke the model, Mauricio Dubon’s MLEs really have to give us pause. His 2019 MLE is non-meaningfully higher than Jacob Heyward’s and Heyward actually projects to a slightly better peak outcome. For a guy who is a really important part of the Giants’ future, that’s a bit worrisome. Dubon was coming off an injury last year which is part of the context the model is missing, but it’s still something to keep an eye on.
Will Wilson’s debut was — statistically speaking — somewhat disastrous. Here’s where overstating the usefulness of these tools can get you into danger. We know Wilson scored very well on the Giants’ draft models, he performed well at a young age in the ACC and he was a consensus top round of the first half pick. Let’s give him a mulligan! Still, woof! was that a bad debut!
Hunter Bishop’s debut wasn’t nearly as bad, but he is clearly separated from the rest of the top prospect group with his 2019 performance. Like Wilson he comes with the caveat of having played a full spring NCAA season prior to pro ball and having shut down for an extended period between those two seasons.
And finally, Marco Luciano can’t get here soon enough! While the MLE calculator doesn’t see him holding his own at the major league level at 17 the way it does with Canario’s performance, it is clearly projecting him to be a superstar with an expected peak production of .404 wOBA. Only 8 players in the majors produced wOBA’s of .400 or above last year and trust me — you know their names.
Rosenblum’s calculator isn’t the only projection model that’s in love with Luciano either:
That seems like a good place to stop for today, but I encourage you to dive into some of these tools as well and see what you can find. I’m very new to playing with data and I’d say my future value as a statistical modeler is probably about a 20 on the 20-80 scale (as you can probably tell from my R exploits above) but the internet is exploding with fascinating new ways to look at the players we’re following and every one is a new piece of the puzzle that is prospecting. What do you think of this model (or others)?
On this Date in History
The last lineup challenge was, of course, the 2005 San Jose Giants who walked it off against Visalia 4-3. Shoutout to Farm Director Kyle Haines’ 1 for 4 with a RBI!
Guess the team and the season (someone break Jeff’s victory streak, please!):
Duggar, CF
Arroyo, 3b
Hinojosa, SS
Shaw, DH
Cole, RF
Jones, R., 1b
Bednar, 2b
Lerud, C
Moncrief, LF
Coonrod, SP
2005: Dan Ortmeier blasted his 16th homer of the year in helping Norwich to a rain-shortened 6-4 victory over Erie. The one-time college teammate of Hunter Pence was enjoying a breakout campaign in his second season in AA. After having injured a shoulder making a diving catch in 2004, he showed that his power had returned with a 20 HR campaign in 2005. Despite playing in by far the worst hitting environment in the Eastern League, Ortmeier produced a top 15 OPS (.853) and was 10th in the league in HRs, just behind Jose Bautista. On September 5 of that year, he’d make his major league debut.
2014: Rafael Rodriguez’ 8th inning, game-tying Grand Slam helped Augusta pull off an audacious come-from-behind extra-inning victory over Kannapolis. The Greenjackets had trailed 6-0 entering the 7th inning. In the 2008, the Giants had made Rodriguez a stunning $2.55 million dollar offer, with club officials including Felipe Alou comparing him to a young Vladimir Guerrero. But, like Angel Villalona before him, RafRod would fail to deliver on the big bonus investment. In this, his third season in Augusta, RafRod would deliver a career high in HRs (5) and produce just a .666 OPS. Halfway through the 2015 season (in which he played sparingly in San Jose) Rodriguez would announce his retirement.
2019: Hunter Bishop showed off his extraordinary batting eye drawing 5 walks in what proved to be his final game in the AZL. Though Bishop’s debut didn’t quite live up to hopes (as seen above), one thing he definitely showed was an extremely patient approach at the plate. During the course of the summer he walked in more than a quarter of his Plate Appearances in the AZL and NWL, piling up 38 walks in just 32 games in his debut. He also once hit a ball 110 MPH btw!
Looks like Richmond 2016.